(Re)in Summary
• Emerging AI innovations are introducing new risks for insurers to deal with.
• Having the right organisational structure and technology is key to addressing these risks.
• Hallucination risk and unintended bias are emerging as the most worrying dangers.
• Failure to align AI systems with regulation is becoming more expensive.
• Keeping a human in the chain is very important to avoid costly AI mistakes.
Insurers in APAC – as elsewhere – are in the midst of an artificial intelligence frenzy, trying to work out the best way to deploy the new technology within their business. But without careful planning and robust governance frameworks, companies are in danger of introducing new risks that weren’t there before.
There are many dangers of AI being used incorrectly. Learning algorithms could produce incorrect or suboptimal decisions, resulting in unintended harm for the insurer or its customers. Poor system implementation may lead to data privacy breaches, with sensitive customer information being exposed. Bias could creep into the new platforms, resulting in unfair treatment of individuals based on the data they are trained on.
“When organizations think about building AI platforms, they need to have in place the capabilities to make sure that all the aspects of compliance, risk and legal are being fairly and effectively dealt with,” says Violet Chung, a Senior Partner with McKinsey & Company in Hong Kong. “This is a very serious issue that must not be overlooked.”
While many large global insurers claim to be on top of the problem, a recent report from McKinsey suggests that most Asian insurers still cling to “traditional organisational structures with multiple intermediaries and limited in-house tech”. This could undermine risk mitigation efforts, says the report.
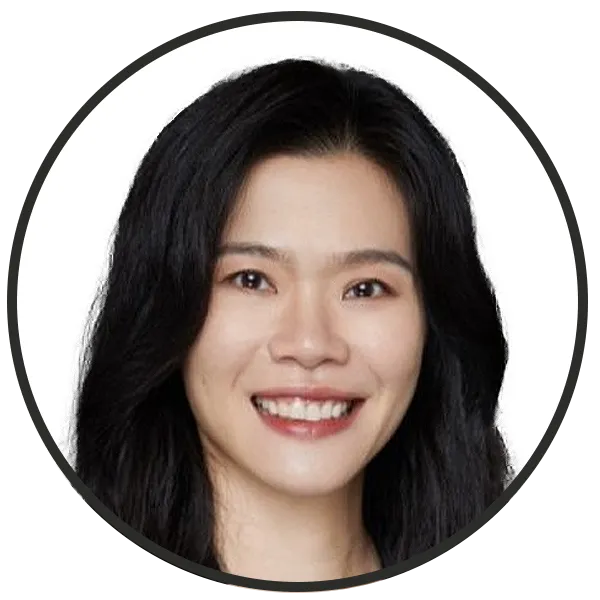
Violet Chung
Senior Partner at McKinsey & CompanyThe importance of good governance
Zurich Insurance Group, which runs its AI innovation hub out of Switzerland, says there are two main aspects to thinking about internal AI risk.
“On one side, there are AI-specific risk triggers, such as model risk, human agency, and recently, risks related to foundational models. On the other side, there are the risks that can arise if these triggers materialise, namely reputational, regulatory and financial risks,” says Matt Reilly, Chief Operations Officer, APAC at Zurich Insurance Group.
Under this framework, all AI innovations that Zurich develops are first put through an impact assessment to calculate a risk score. Factors such as model complexity, the classification of data being processed and the human impact (on both customers and employees) are all considered in determining the risk score. This then informs the AI governance requirements that are applied.
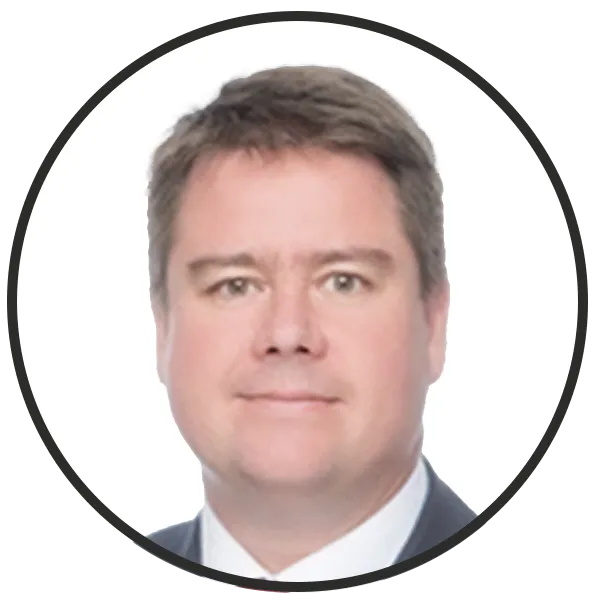
Matt Reilly
Chief Operations Officer for APAC at Zurich Insurance Group“[Our] approach to AI and data underscores a balance between leveraging cutting-edge technologies for operational efficiency and customer service while adhering to strict ethical, privacy and security standards,” says Reilly.
Zurich has been piloting a number of AI projects around the world, including in Asia. The Swiss-headquartered company is particularly interested in cultivating natural language models to improve claims automation, and in using AI to enhance customer experience.
Zurich has spent the past five years developing its own internal AI assurance framework, building on data integrity practices that it had already put in place.
Hallucination risks and unintended bias
One of the most worrying risks associated with AI systems, and one that may be the hardest to handle, is what Chung refers to as ‘hallucination risk’. This is the possibility that an AI algorithm produces output that everyone assumes is correct when in actual fact it isn’t.
“Generative AI is very good at using multiple inputs to derive single output, but it is vital that insurers can trust the AI models they are working with. Firms don’t want their AI models to come up with unfactual facts, especially when applied to real business scenarios and real customers,” says Chung.
With many insurance AI systems seeking to reduce costs and increase efficiency for customers, “the margin of error has to managed with upmost caution”, says Chung.
Related to this is the danger of unintended bias caused by inappropriate data sets. An example of this is where an AI algorithm extrapolates data from one market to say something about another market, without properly accounting for the local context.
“This is about knowing the provenance of the data, and selecting the right data models depending on how and where the AI system is being deployed,” says Winston Yong, an Enterprise Architect for Technology Strategy at IBM Consulting. “For example, if you have an AI platform that has been developed in the United States but trained on data from China, then you will have a China bias that may not work elsewhere.”
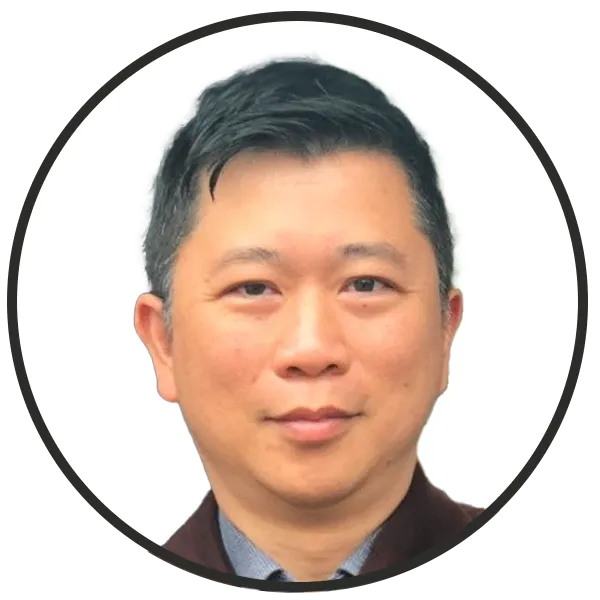
Winston Yong
Enterprise Architect for Technology Strategy at IBM ConsultingPeople and process
The problem with AI algorithms getting things wrong is that, once a mistake has been made, it is often amplified as the system continues to learn from the market environment.
“The bias might start off small, but as the system continues to veer off and veer off, these errors rapidly accumulate,” says Yong.
This is why insurers, like Zurich, have been taking this kind of risk so seriously right from the start.
“The mindset must be focused from day zero on building a robust tech risk management team. This cannot just be considered as an afterthought,” says Chung.
Yong adds that the important thing is for insurers to see AI as augmenting human activity rather than replacing it.
“You need to empower people to improve the role that they are performing, while letting AI do all the heavy lifting,” says Yong.
Violet Chung
Partner at McKinseyKeeping a human in the chain is still very important, he says.
To illustrate the point, Yong uses the example of an AI system that scans photos of car accidents to detect fraudulent claims – something that insurance companies are trialling at the moment. Such an AI system would calculate the likelihood of fraud taking place, and then pass this back to a human to verify the result.
“AI shouldn’t be running independently. It should be there to assist managers in making better decisions,” says Yong.
Matt Reilly
Chief Operations Officer for APAC at Zurich Insurance GroupThis comes back to encasing AI innovations in appropriate governance frameworks.
“Zurich’s approach is to augment human capabilities through AI, rather than replacing them by continuously improving AI accuracy and reliability to exceed human performance standards, particularly in fraud detection, claims processing and underwriting decisions. Zurich aims to enhance human decision-making and reduce the margin of error,” says Reilly.
What constitutes an acceptable margin of error can vary from case-to-case, though.
“There are cases where a higher tolerance to errors is acceptable (such as when AI is used to innovate and be creative without any potential for human harm) and there are cases in which the error needs to be minimized to the greatest extent possible (such as when AI is being used in underwriting),” says Reilly. “We strongly believe that humans and AI together achieve better outcomes than either one on its own.”
Regulation and reputational risk
Beyond model risk, insurers also have to be aware of reputational risk and penalties that they could incur from regulators.
For example, European companies could be fined up to 7% of their global annual turnover for failing to comply with the EU’s new AI law.
APAC jurisdictions have not yet introduced comparable legislation but many are watching carefully how things develop in the EU.
A number of lawsuits have already been filed in Europe and the US for misuse of generative AI. These include a complaint against Microsoft’s GitHub for allegedly mishandling personal data, complaints over alleged copyright infringement by image generator providers Stability AI, Midjourney, and Deviant Art, and a class action lawsuit against Google for misuse of personal data and copyright infringement.
“Inspiring digital trust is a key strategic driver for Zurich, as we handle large volumes of customer data, including sensitive personal data,” says Reilly. “It is key that our customers fully trust us when sharing such information.”